Transforming Extrusion Processes with Advanced Machine Learning
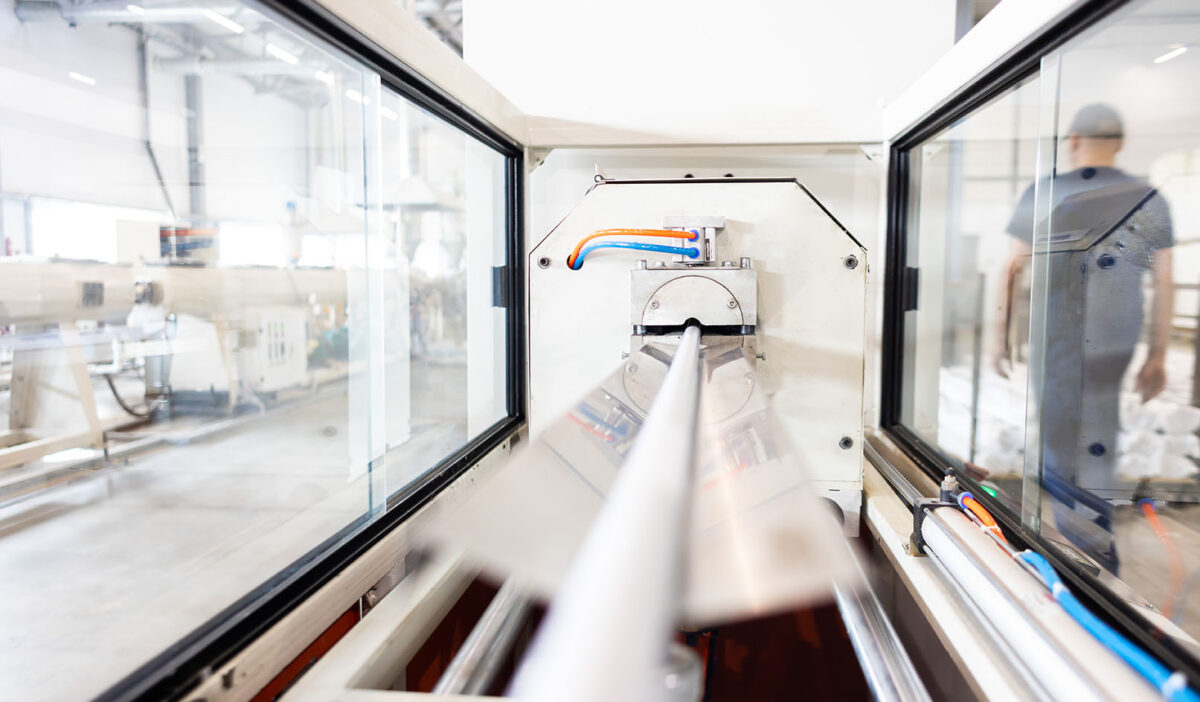
The diagnosis and monitoring of extrusion processes have historically relied on rule-based algorithms. While effective for many years, this conventional approach faces inherent limitations. These limitations include a restricted number of controllable parameters, vulnerability to irrelevant outliers, and heightened complexity in systems with extensive degrees of freedom.
This groundbreaking study marks a significant departure from tradition by introducing machine learning models to diagnose and monitor extrusion processes. Going beyond the confines of rule-based algorithms, this approach incorporates over 80 process variables, offering a comprehensive understanding of the intricate extrusion landscape.
Mahalanobis Distance: A Pioneering Metric
The machine learning model employs the Mahalanobis Distance metric as a foundational tool. This metric enables the establishment of boundaries for stable processing conditions. By leveraging the Mahalanobis Distance, the model gains insights into the intricate relationships between process variables and defines a robust framework for optimal extrusion.
Building upon this foundation, the model dynamically adapts to the variability inherent in extrusion processes. Armed with a deep understanding of stable processing conditions, the model scrutinizes incoming data in real-time. Any deviations from the established stable conditions are promptly identified, allowing for swift corrective measures.
Unleashing the Potential of Extrusion Technology
This innovative application of machine learning not only overcomes the limitations of traditional approaches but also unleashes the full potential of extrusion technology. The incorporation of advanced data analytics in diagnosing and monitoring processes signifies a paradigm shift in the plastics engineering landscape, promising enhanced efficiency, reduced downtime, and optimized extrusion outcomes.
The integration of machine learning models, spearheaded by the Mahalanobis Distance metric, heralds a new era in extrusion processes, offering unprecedented insights and adaptability for the plastics engineering industry.
To learn more on this topic, attend ANTEC 2024 in St. Louis. John W.S. Lee, Principal Research Engineer/Data Scientist, LS Cable & System Ltd. will be presenting, “Enhancing Extrusion Process Diagnosis and Monitoring Through Machine Learning“, on Wednesday, March 6.